Here is a possible solution using shapely.
It generates a shapely Polygon for each cluster hull.
Then, it checks each point in a given dataframe (df1) is within at least one of the hulls.
Plots shows the cluster points (red/blue/orange/cyan), the cluster hulls (dashed lines, same colors), the datapoints to test in black (out of cluster hull) and green (inside cluster hull).
Modules versions:
Python 3.9.13 (tags/v3.9.13:6de2ca5, May 17 2022, 16:36:42) [MSC v.1929 64 bit (AMD64)]
pandas 1.4.1
shapely 1.7.1
numpy 1.22.3
matplotlib 3.5.1
scipy 1.8.1
Matplotlib:
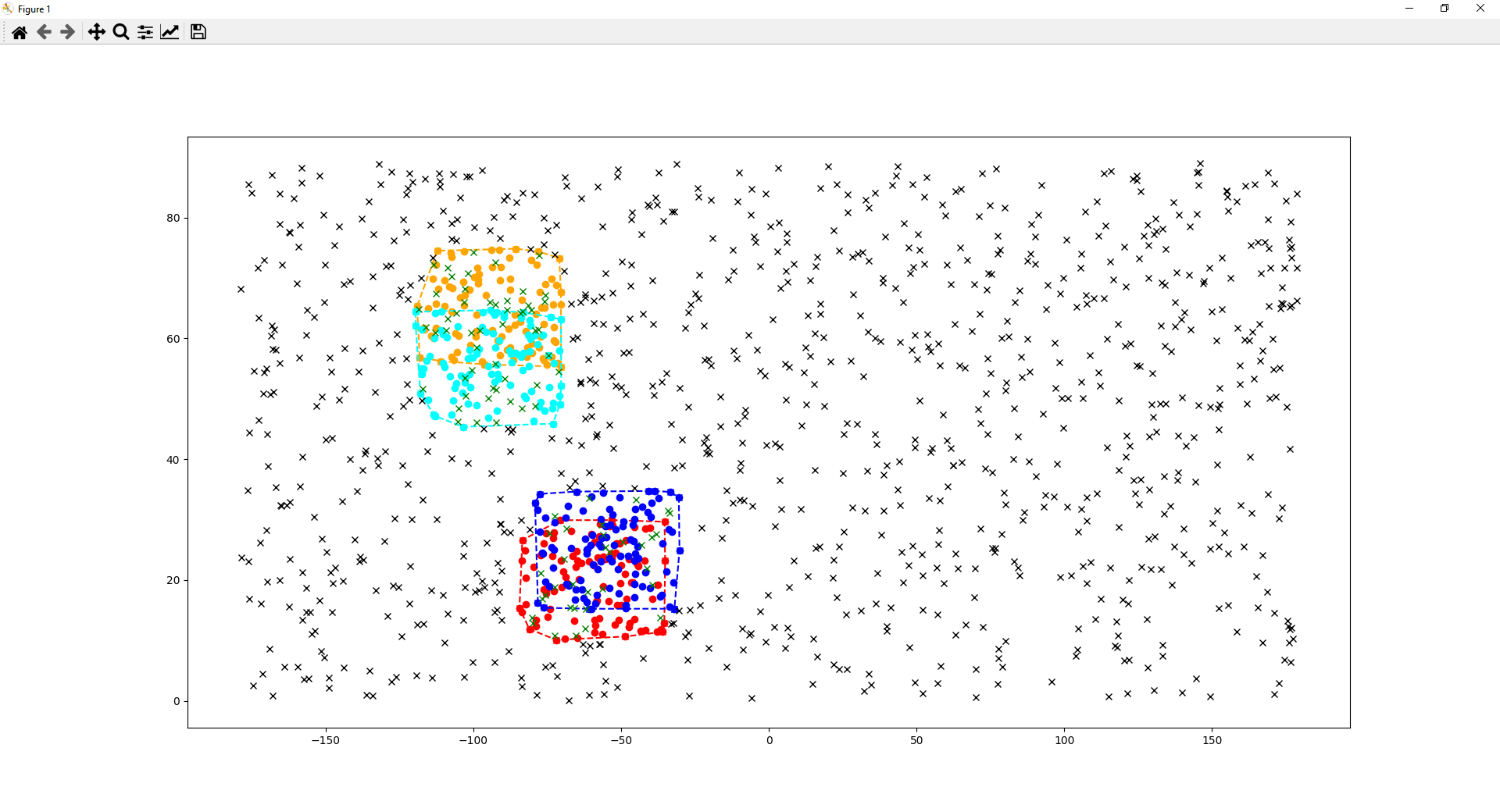
import sys
from typing import List
import matplotlib
import numpy as np
import pandas as pd
import scipy
import shapely
from matplotlib import pyplot as plt
from scipy.spatial import ConvexHull
from shapely.geometry import Point, Polygon
print("Python", sys.version)
for m in [pd, shapely, np, matplotlib, scipy]:
print(m.__name__, m.__version__)
def generate_random(n: int,
low_lat: int, high_lat: int,
low_lon: int, high_lon: int,
cluster_id: int):
cols = ["latitude", "longitude"]
if cluster_id:
cols.append("cluster")
df = pd.DataFrame(columns=cols)
df.latitude = np.random.uniform(low=low_lat, high=high_lat, size=(n,))
df.longitude = np.random.uniform(low=low_lon, high=high_lon, size=(n,))
if cluster_id:
df.cluster = cluster_id
return df
def coords_to_shapely_point(x: np.ndarray, y: np.ndarray) -> List[Point]:
return list(map(Point, x.tolist(), y.tolist()))
def generate_random_inputs():
nb_clusters_to_generate = 4
df_clusterrs = []
for i in range(nb_clusters_to_generate):
lat_offset = int(np.random.choice(a=[-25, -20, -15, 15, 20, 25], size=(1,)))
lon_offset = int(np.random.choice(a=[-25, -20, -15, 15, 20, 25], size=(1,)))
df_clutser_i = generate_random(n=100, cluster_id=i + 1, # stort from 1, 0 = no cluster
low_lat=30 + lat_offset, high_lat=50 + lat_offset,
low_lon=-100 + lon_offset, high_lon=-50 + lon_offset)
df_clusterrs.append(df_clutser_i)
df = pd.concat(df_clusterrs)
df1 = generate_random(n=1000, cluster_id=0, low_lat=0, high_lat=89, low_lon=-179, high_lon=180)
return df, df1
df, df1 = generate_random_inputs()
cluster_geoms = []
for color, cluster_id in zip(["red", "blue", "orange", "cyan"],
df.cluster.unique().tolist()):
dfq = df[df["cluster"] == cluster_id]
df_cluster_coords = dfq[["latitude", "longitude"]]
Y = df_cluster_coords.to_numpy()
# plot cluster data
plt.plot(Y[:, 1], Y[:, 0], 'o', color=color)
# compute hull
hull = ConvexHull(Y)
# hull.vertices gives indexes of the hull points
hull_vertices = df_cluster_coords.iloc[hull.vertices].to_numpy()
_hull_X = hull_vertices[:, 1]
_hull_Y = hull_vertices[:, 0]
# close shape
_hull_X = np.append(_hull_X, _hull_X[0])
_hull_Y = np.append(_hull_Y, _hull_Y[0])
plt.plot(_hull_X, _hull_Y, "x", color=color, ls="--")
cluster_geoms.append(Polygon(coords_to_shapely_point(x=_hull_X, y=_hull_Y)))
def is_in_clusters(row, clusters: List[Polygon]) -> bool:
p = Point(row.longitude, row.latitude)
is_in_cluster = False
for c_geom in clusters:
is_in_cluster |= c_geom.contains(p)
return is_in_cluster
df1["in_cluster"] = df1.apply(is_in_clusters, axis=1, clusters=cluster_geoms)
for latitude, longitude, in_cluster in df1.itertuples(index=False):
plt.plot(longitude, latitude, "x", color="green" if in_cluster else "black")
plt.show()